When typing this question into ChatGPT I was impressed by the response that suggests deep insight into the relationship between the shape, weight and strength of a manhole cover, the challenges to manufacture and manipulate it as well as worker safety concerns. Surely an AI needs a model of the world and true reasoning capability to arrive at this answer?
But is this real reasoning, or is there less than meets the eye? It turns out that, through an intricate dance of data retrieval and pattern recognition the large language model is constructing its answer from the countless internet pages in its dataset where this HR interview question is discussed in detail. Many examples of LLM ‘intelligence’ can similarly be traced back to the dataset and the response accuracy drops immensely when we
· reduce its ability to search its dataset, for example by changing the terminology used to describe the problem or
· propose problems outside of the dataset
The same is true when trying to solve planning problems where the LLM will be ‘approximately retrieving’ a plan from the many plans it has seen in its gigantic dataset. This is not reasoning but actually still very useful, as long as we have a way to validate these generated ‘candidate plans’, either by human experts or reliable planning software.
We can create significant business value with this technology as long as we
· don’t get carried away by the hype and
· understand that we are very much in the man + machine era
So why are manhole covers round? Ask your favourite LLM!
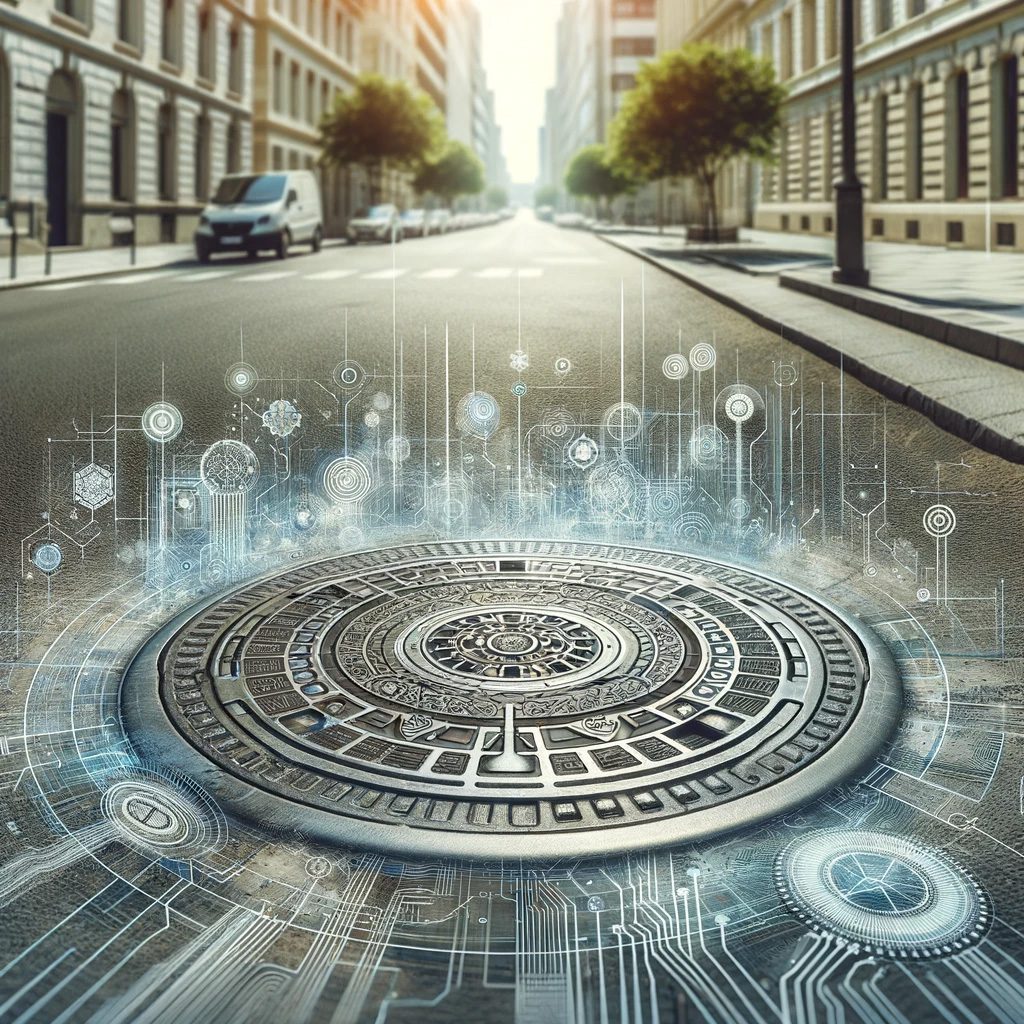